Denodo Datafest NYC has concluded with excellent talks and discussions around data virtualization, real-time data APIs, information fabric, data catalogs, and real-world big data problems. It was great meeting and speaking to technologists and business professionals like Brian Hopkins, Michele Goetz, Luke Slotwinski, Mike Sofen, Nicolas Brisoux, Madeleine Corneli, Avinash Deshpande, Paco Hernandez, Kurt Jackson, Arif Rajwani, Mano Vajpey, Vincent L'Archeveque, Minnix, Rajat Sinha, Vineet Talwar, Steve Young, Steven Leistner, Ignacio, Ravi Shankar and many others.
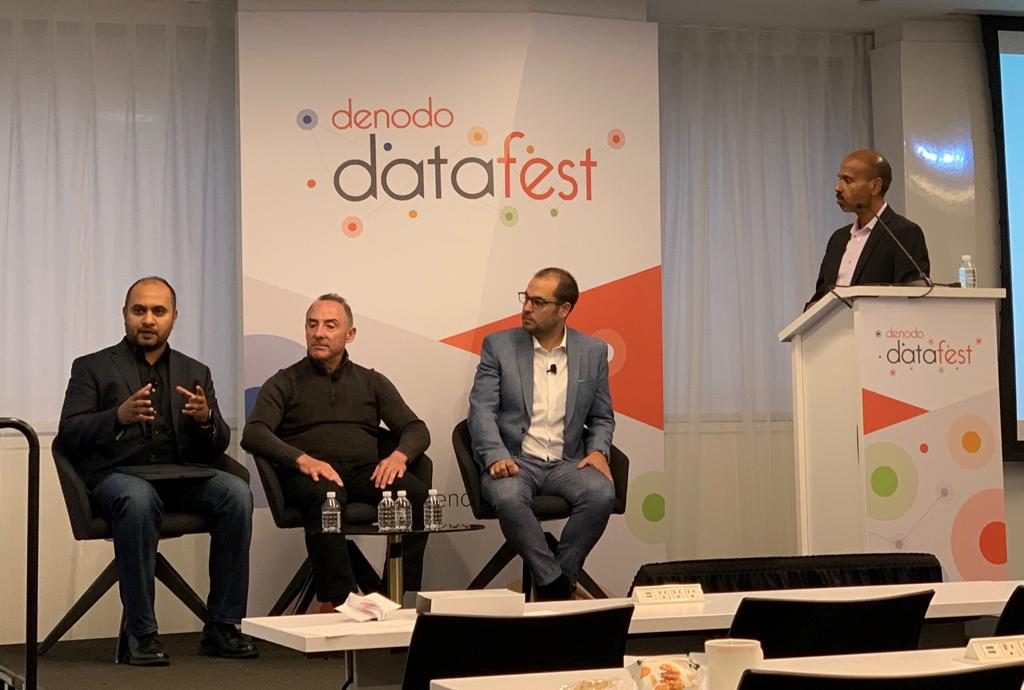
I had the distinct pleasure to speak to the audience about data science bottlenecks i.e. how real data science use cases unfold when the MLOps lifecycle becomes critical -- case studies like training a faster RCNN Model for detecting lab equipments, doing customer escalation triage probability analysis from complaint dataset, using attention network for machine comprehension in contract analysis for keyterms in LIBOR, building meaningful healthcare ontologies to respond to realtime queries, working with pretrained vgg6 model for roof age and condition detection etc. In essence, data Science roadblocks span the entire lifecycle.
For those of us who have toiled beyond hello-world projects in the enterprise, we know this very well that getting meaningful data is hard in presence of data silos and data lake catch-22s. Data virtualization helps provide fast results by integrating disparate data from any enterprise source, big data and cloud in real time. The approach is different from traditional ETL solutions, which replicate data, data virtualization leaves the data in source systems, simply exposing an integrated view of all the data to data consumers. From a data science perspective, data virtualization helps by providing a logical data layer that integrates all enterprise data siloed across the disparate systems, building the Information Fabric.
I believe the data challenges can be divided into 3 main categories -- with virtualization, MLOps, and explainability each solving part of the puzzle.
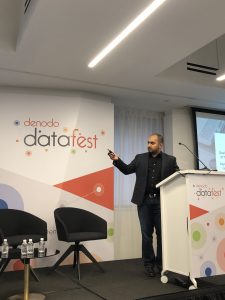
Availability Challenges
- Data silos, modalities, fragmented - Cleaning
- Data quality and amount of training data
- Ingestion, analysis, transformation, training, serving, Model Development
Continuity Challenges
- Deployment / operationalization
- Delivery of accurate models with consideration for model and data drift
- Relevant Business Accuracy measures
- Versioning of data and models for governance
and finally
Explainability Challenges
- Interpetabilty and Explainability
- Reproduciblity
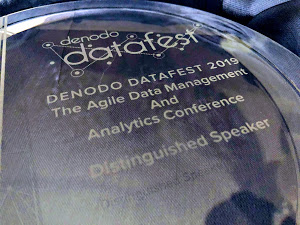